Cost function for LTI system identification
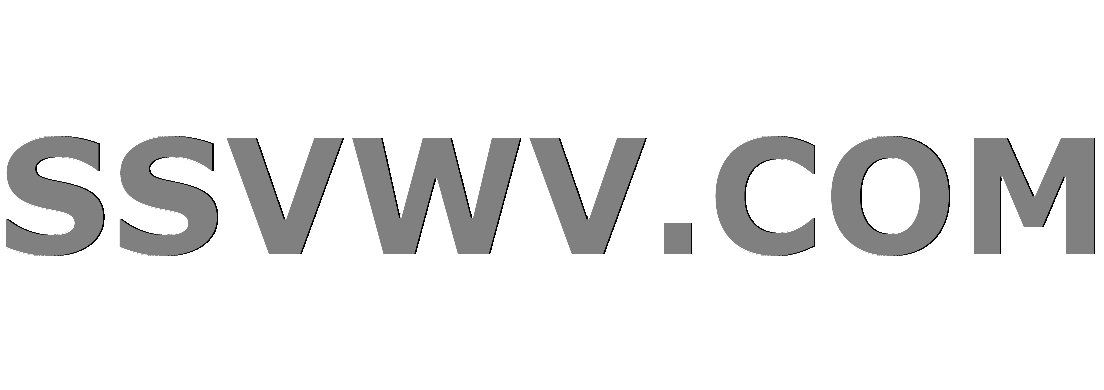
Multi tool use
$begingroup$
I am currently reading and trying to understand a paper (Kulkarni and Colburn, 2004) that utilizes system identification methods to approximate head-related transfer functions.
The general approach is to
- Compute an autoregressive (all-pole-) estimate of the transfer function using the autocorrelation method for linear prediction.
- Use the AR estimate as a starting point to compute a pole-zero-representation of the system transfer function iteratively.
- Evaluate the result of the estimation process on a logarithmic scale (Error in dB).
For the iterative procedure, the authors are proposing a cost function
$hat{C} = frac{1}{2pi}int_{-pi}^{pi}|H(e^{jomega})A(e^{jomega}) - B(e^{jomega})|^2 domega $,
where $hat{H}$ is the system transfer function, $A$ is the DTFT of the recursive coefficients and $B$ the DTFT of the transversal coefficients.
I understand this approach originates from this paper (Kalman, 1958).
This cost function is then extended for the iterative process as
$hat{C}_i = frac{1}{2pi}int_{-pi}^{pi}|frac{H(e^{jomega})A_i(e^{jomega})}{A_{i-1}(e^{jomega})} - frac{B_i(e^{jomega})}{A_{i-1}(e^{jomega})}|^2 domega $,
where the index $i$ denotes variables corresponding to the $i$th iteration.
The iterative modification originates from this paper (Steiglitz and McBride, 1965).
In order to find a solution on a decibel scale, a weighting function $W$ is introduced:
$hat{C}_i = frac{1}{2pi}int_{-pi}^{pi} |W_i(e^{jomega})|^2 |frac{H(e^{jomega})A_i(e^{jomega})}{A_{i-1}(e^{jomega})} - frac{B_i(e^{jomega})}{A_{i-1}(e^{jomega})}|^2 domega $,
which is introduced in the paper as
$W_i(e^{jomega}) = frac{logleft( H(e^{jomega})right) - logleft( frac{B_{i-1}(e^{jomega})}{A_{i-1}(e^{jomega})}right)}{|H(e^{jomega})A_{i-1}(e^{jomega}) - B_{i-1}(e^{jomega})|^2}$.
I understand this weighting function is the squared error in logarithmic scale between true and approximated transfer function, divided by the first cost function.
However, i have trouble understanding the process of arriving at the iterative cost function for several reasons. I would like to ask the following questions:
- Why is the first cost function $hat{C}$ preferred to, say, $frac{1}{2pi}int_{-pi}^{pi} |H(e^{jomega}) - B(e^{jomega})/A(e^{jomega})|^2$ in the first place? what does it do?
- In the iterative cost function $hat{C}_i$, what is the purpose of dividing by the recursive proportion of the last transfer function estimate?
- For what reason is a weighting function introduced for logarithmic error minimization, rather than just using its numerator as a cost function directly?
I would really appreciate any help or pointers into the right direction.
linear-systems transfer-function system-identification autoregressive-model
$endgroup$
add a comment |
$begingroup$
I am currently reading and trying to understand a paper (Kulkarni and Colburn, 2004) that utilizes system identification methods to approximate head-related transfer functions.
The general approach is to
- Compute an autoregressive (all-pole-) estimate of the transfer function using the autocorrelation method for linear prediction.
- Use the AR estimate as a starting point to compute a pole-zero-representation of the system transfer function iteratively.
- Evaluate the result of the estimation process on a logarithmic scale (Error in dB).
For the iterative procedure, the authors are proposing a cost function
$hat{C} = frac{1}{2pi}int_{-pi}^{pi}|H(e^{jomega})A(e^{jomega}) - B(e^{jomega})|^2 domega $,
where $hat{H}$ is the system transfer function, $A$ is the DTFT of the recursive coefficients and $B$ the DTFT of the transversal coefficients.
I understand this approach originates from this paper (Kalman, 1958).
This cost function is then extended for the iterative process as
$hat{C}_i = frac{1}{2pi}int_{-pi}^{pi}|frac{H(e^{jomega})A_i(e^{jomega})}{A_{i-1}(e^{jomega})} - frac{B_i(e^{jomega})}{A_{i-1}(e^{jomega})}|^2 domega $,
where the index $i$ denotes variables corresponding to the $i$th iteration.
The iterative modification originates from this paper (Steiglitz and McBride, 1965).
In order to find a solution on a decibel scale, a weighting function $W$ is introduced:
$hat{C}_i = frac{1}{2pi}int_{-pi}^{pi} |W_i(e^{jomega})|^2 |frac{H(e^{jomega})A_i(e^{jomega})}{A_{i-1}(e^{jomega})} - frac{B_i(e^{jomega})}{A_{i-1}(e^{jomega})}|^2 domega $,
which is introduced in the paper as
$W_i(e^{jomega}) = frac{logleft( H(e^{jomega})right) - logleft( frac{B_{i-1}(e^{jomega})}{A_{i-1}(e^{jomega})}right)}{|H(e^{jomega})A_{i-1}(e^{jomega}) - B_{i-1}(e^{jomega})|^2}$.
I understand this weighting function is the squared error in logarithmic scale between true and approximated transfer function, divided by the first cost function.
However, i have trouble understanding the process of arriving at the iterative cost function for several reasons. I would like to ask the following questions:
- Why is the first cost function $hat{C}$ preferred to, say, $frac{1}{2pi}int_{-pi}^{pi} |H(e^{jomega}) - B(e^{jomega})/A(e^{jomega})|^2$ in the first place? what does it do?
- In the iterative cost function $hat{C}_i$, what is the purpose of dividing by the recursive proportion of the last transfer function estimate?
- For what reason is a weighting function introduced for logarithmic error minimization, rather than just using its numerator as a cost function directly?
I would really appreciate any help or pointers into the right direction.
linear-systems transfer-function system-identification autoregressive-model
$endgroup$
add a comment |
$begingroup$
I am currently reading and trying to understand a paper (Kulkarni and Colburn, 2004) that utilizes system identification methods to approximate head-related transfer functions.
The general approach is to
- Compute an autoregressive (all-pole-) estimate of the transfer function using the autocorrelation method for linear prediction.
- Use the AR estimate as a starting point to compute a pole-zero-representation of the system transfer function iteratively.
- Evaluate the result of the estimation process on a logarithmic scale (Error in dB).
For the iterative procedure, the authors are proposing a cost function
$hat{C} = frac{1}{2pi}int_{-pi}^{pi}|H(e^{jomega})A(e^{jomega}) - B(e^{jomega})|^2 domega $,
where $hat{H}$ is the system transfer function, $A$ is the DTFT of the recursive coefficients and $B$ the DTFT of the transversal coefficients.
I understand this approach originates from this paper (Kalman, 1958).
This cost function is then extended for the iterative process as
$hat{C}_i = frac{1}{2pi}int_{-pi}^{pi}|frac{H(e^{jomega})A_i(e^{jomega})}{A_{i-1}(e^{jomega})} - frac{B_i(e^{jomega})}{A_{i-1}(e^{jomega})}|^2 domega $,
where the index $i$ denotes variables corresponding to the $i$th iteration.
The iterative modification originates from this paper (Steiglitz and McBride, 1965).
In order to find a solution on a decibel scale, a weighting function $W$ is introduced:
$hat{C}_i = frac{1}{2pi}int_{-pi}^{pi} |W_i(e^{jomega})|^2 |frac{H(e^{jomega})A_i(e^{jomega})}{A_{i-1}(e^{jomega})} - frac{B_i(e^{jomega})}{A_{i-1}(e^{jomega})}|^2 domega $,
which is introduced in the paper as
$W_i(e^{jomega}) = frac{logleft( H(e^{jomega})right) - logleft( frac{B_{i-1}(e^{jomega})}{A_{i-1}(e^{jomega})}right)}{|H(e^{jomega})A_{i-1}(e^{jomega}) - B_{i-1}(e^{jomega})|^2}$.
I understand this weighting function is the squared error in logarithmic scale between true and approximated transfer function, divided by the first cost function.
However, i have trouble understanding the process of arriving at the iterative cost function for several reasons. I would like to ask the following questions:
- Why is the first cost function $hat{C}$ preferred to, say, $frac{1}{2pi}int_{-pi}^{pi} |H(e^{jomega}) - B(e^{jomega})/A(e^{jomega})|^2$ in the first place? what does it do?
- In the iterative cost function $hat{C}_i$, what is the purpose of dividing by the recursive proportion of the last transfer function estimate?
- For what reason is a weighting function introduced for logarithmic error minimization, rather than just using its numerator as a cost function directly?
I would really appreciate any help or pointers into the right direction.
linear-systems transfer-function system-identification autoregressive-model
$endgroup$
I am currently reading and trying to understand a paper (Kulkarni and Colburn, 2004) that utilizes system identification methods to approximate head-related transfer functions.
The general approach is to
- Compute an autoregressive (all-pole-) estimate of the transfer function using the autocorrelation method for linear prediction.
- Use the AR estimate as a starting point to compute a pole-zero-representation of the system transfer function iteratively.
- Evaluate the result of the estimation process on a logarithmic scale (Error in dB).
For the iterative procedure, the authors are proposing a cost function
$hat{C} = frac{1}{2pi}int_{-pi}^{pi}|H(e^{jomega})A(e^{jomega}) - B(e^{jomega})|^2 domega $,
where $hat{H}$ is the system transfer function, $A$ is the DTFT of the recursive coefficients and $B$ the DTFT of the transversal coefficients.
I understand this approach originates from this paper (Kalman, 1958).
This cost function is then extended for the iterative process as
$hat{C}_i = frac{1}{2pi}int_{-pi}^{pi}|frac{H(e^{jomega})A_i(e^{jomega})}{A_{i-1}(e^{jomega})} - frac{B_i(e^{jomega})}{A_{i-1}(e^{jomega})}|^2 domega $,
where the index $i$ denotes variables corresponding to the $i$th iteration.
The iterative modification originates from this paper (Steiglitz and McBride, 1965).
In order to find a solution on a decibel scale, a weighting function $W$ is introduced:
$hat{C}_i = frac{1}{2pi}int_{-pi}^{pi} |W_i(e^{jomega})|^2 |frac{H(e^{jomega})A_i(e^{jomega})}{A_{i-1}(e^{jomega})} - frac{B_i(e^{jomega})}{A_{i-1}(e^{jomega})}|^2 domega $,
which is introduced in the paper as
$W_i(e^{jomega}) = frac{logleft( H(e^{jomega})right) - logleft( frac{B_{i-1}(e^{jomega})}{A_{i-1}(e^{jomega})}right)}{|H(e^{jomega})A_{i-1}(e^{jomega}) - B_{i-1}(e^{jomega})|^2}$.
I understand this weighting function is the squared error in logarithmic scale between true and approximated transfer function, divided by the first cost function.
However, i have trouble understanding the process of arriving at the iterative cost function for several reasons. I would like to ask the following questions:
- Why is the first cost function $hat{C}$ preferred to, say, $frac{1}{2pi}int_{-pi}^{pi} |H(e^{jomega}) - B(e^{jomega})/A(e^{jomega})|^2$ in the first place? what does it do?
- In the iterative cost function $hat{C}_i$, what is the purpose of dividing by the recursive proportion of the last transfer function estimate?
- For what reason is a weighting function introduced for logarithmic error minimization, rather than just using its numerator as a cost function directly?
I would really appreciate any help or pointers into the right direction.
linear-systems transfer-function system-identification autoregressive-model
linear-systems transfer-function system-identification autoregressive-model
edited 12 mins ago
Jonas Schwarz
asked 1 hour ago
Jonas SchwarzJonas Schwarz
262112
262112
add a comment |
add a comment |
1 Answer
1
active
oldest
votes
$begingroup$
The chosen cost function is the mean squared error, i.e., the integral over a squared magnitude of the difference between frequency responses. The function
$$E(e^{jomega})=H(e^{jomega})-frac{B(e^{jomega})}{A(e^{j omega})}tag{1}$$
depends on frequency, so you can't minimize it directly, unless you want to minimize it for exactly one frequency $omega$, which is of course pointless. You can choose several error measures depending on $E(e^{jomega})$ given in $(1)$. Two common choices are
$$varepsilon_1=max_{omega}W(omega)|E(e^{jomega})|tag{2}$$
and
$$varepsilon_2=int_{0}^{pi}W(omega)|E(e^{jomega})|^2domegatag{3}$$
with some positive weighting function $W(omega)$. Note that $varepsilon_1$ and $varepsilon_2$ given by $(2)$ and $(3)$ do not depend on frequency.
The authors of the paper you refer to chose to minimize the weighted mean square error given by $(3)$. However, instead of using the linear difference $(1)$, they chose to minimize the average logarithmic difference, i.e., the average error on a dB scale.
The problem with the minimization of $(3)$ is that for IIR filters, it results in a non-linear optimization problem, which is much harder to solve directly, and which might also have locally optimal solutions that are far from the global optimum. The cost function $hat{C}$ in your question is linear in the filter coefficients. The point of the iteration is now to solve a sequence of linear minimization problems (which is simple, just solve a system of linear equations) in order to compute the solution of the originally non-linear optimization problem (the minimization of $(3)$). Note that if convergence is achieved, then $A_{i-1}(e^{jomega})=A_{i}(e^{jomega})$, so the cost function $hat{C}_i$ is identical to the cost function of the original non-linear problem. Yet, only linear minimization problems are solved in each iteration.
I'm not sure I completely understand your last question, but the weighting function is there to change to problem from minimizing the average squared difference between frequency responses to the average squared differences between the logarithms of the magnitude responses. The necessary weighting function is unknown, but if the procedure converges - note that there is no guarantee that it does in all cases - the final weight function is such that the mean squared logarithmic error is minimized. Note that this latter problem is highly non-linear, whereas the proposed procedure only solves linear subproblems.
$endgroup$
$begingroup$
Thank you for your response! I now understand why the weighting is utilized. Could you elaborate on what is achieved by dividing by $A_{i-1}$ in the iterative cost function? Does it force convergence?
$endgroup$
– Jonas Schwarz
14 mins ago
$begingroup$
Also, i made an error in my first question, i meant to ask about the integral over the difference of true and estimated transfer function instead of a frequency-dependent error. I'll edit the question.
$endgroup$
– Jonas Schwarz
14 mins ago
add a comment |
Your Answer
StackExchange.ready(function() {
var channelOptions = {
tags: "".split(" "),
id: "295"
};
initTagRenderer("".split(" "), "".split(" "), channelOptions);
StackExchange.using("externalEditor", function() {
// Have to fire editor after snippets, if snippets enabled
if (StackExchange.settings.snippets.snippetsEnabled) {
StackExchange.using("snippets", function() {
createEditor();
});
}
else {
createEditor();
}
});
function createEditor() {
StackExchange.prepareEditor({
heartbeatType: 'answer',
autoActivateHeartbeat: false,
convertImagesToLinks: false,
noModals: true,
showLowRepImageUploadWarning: true,
reputationToPostImages: null,
bindNavPrevention: true,
postfix: "",
imageUploader: {
brandingHtml: "Powered by u003ca class="icon-imgur-white" href="https://imgur.com/"u003eu003c/au003e",
contentPolicyHtml: "User contributions licensed under u003ca href="https://creativecommons.org/licenses/by-sa/3.0/"u003ecc by-sa 3.0 with attribution requiredu003c/au003e u003ca href="https://stackoverflow.com/legal/content-policy"u003e(content policy)u003c/au003e",
allowUrls: true
},
noCode: true, onDemand: true,
discardSelector: ".discard-answer"
,immediatelyShowMarkdownHelp:true
});
}
});
Sign up or log in
StackExchange.ready(function () {
StackExchange.helpers.onClickDraftSave('#login-link');
});
Sign up using Google
Sign up using Facebook
Sign up using Email and Password
Post as a guest
Required, but never shown
StackExchange.ready(
function () {
StackExchange.openid.initPostLogin('.new-post-login', 'https%3a%2f%2fdsp.stackexchange.com%2fquestions%2f56860%2fcost-function-for-lti-system-identification%23new-answer', 'question_page');
}
);
Post as a guest
Required, but never shown
1 Answer
1
active
oldest
votes
1 Answer
1
active
oldest
votes
active
oldest
votes
active
oldest
votes
$begingroup$
The chosen cost function is the mean squared error, i.e., the integral over a squared magnitude of the difference between frequency responses. The function
$$E(e^{jomega})=H(e^{jomega})-frac{B(e^{jomega})}{A(e^{j omega})}tag{1}$$
depends on frequency, so you can't minimize it directly, unless you want to minimize it for exactly one frequency $omega$, which is of course pointless. You can choose several error measures depending on $E(e^{jomega})$ given in $(1)$. Two common choices are
$$varepsilon_1=max_{omega}W(omega)|E(e^{jomega})|tag{2}$$
and
$$varepsilon_2=int_{0}^{pi}W(omega)|E(e^{jomega})|^2domegatag{3}$$
with some positive weighting function $W(omega)$. Note that $varepsilon_1$ and $varepsilon_2$ given by $(2)$ and $(3)$ do not depend on frequency.
The authors of the paper you refer to chose to minimize the weighted mean square error given by $(3)$. However, instead of using the linear difference $(1)$, they chose to minimize the average logarithmic difference, i.e., the average error on a dB scale.
The problem with the minimization of $(3)$ is that for IIR filters, it results in a non-linear optimization problem, which is much harder to solve directly, and which might also have locally optimal solutions that are far from the global optimum. The cost function $hat{C}$ in your question is linear in the filter coefficients. The point of the iteration is now to solve a sequence of linear minimization problems (which is simple, just solve a system of linear equations) in order to compute the solution of the originally non-linear optimization problem (the minimization of $(3)$). Note that if convergence is achieved, then $A_{i-1}(e^{jomega})=A_{i}(e^{jomega})$, so the cost function $hat{C}_i$ is identical to the cost function of the original non-linear problem. Yet, only linear minimization problems are solved in each iteration.
I'm not sure I completely understand your last question, but the weighting function is there to change to problem from minimizing the average squared difference between frequency responses to the average squared differences between the logarithms of the magnitude responses. The necessary weighting function is unknown, but if the procedure converges - note that there is no guarantee that it does in all cases - the final weight function is such that the mean squared logarithmic error is minimized. Note that this latter problem is highly non-linear, whereas the proposed procedure only solves linear subproblems.
$endgroup$
$begingroup$
Thank you for your response! I now understand why the weighting is utilized. Could you elaborate on what is achieved by dividing by $A_{i-1}$ in the iterative cost function? Does it force convergence?
$endgroup$
– Jonas Schwarz
14 mins ago
$begingroup$
Also, i made an error in my first question, i meant to ask about the integral over the difference of true and estimated transfer function instead of a frequency-dependent error. I'll edit the question.
$endgroup$
– Jonas Schwarz
14 mins ago
add a comment |
$begingroup$
The chosen cost function is the mean squared error, i.e., the integral over a squared magnitude of the difference between frequency responses. The function
$$E(e^{jomega})=H(e^{jomega})-frac{B(e^{jomega})}{A(e^{j omega})}tag{1}$$
depends on frequency, so you can't minimize it directly, unless you want to minimize it for exactly one frequency $omega$, which is of course pointless. You can choose several error measures depending on $E(e^{jomega})$ given in $(1)$. Two common choices are
$$varepsilon_1=max_{omega}W(omega)|E(e^{jomega})|tag{2}$$
and
$$varepsilon_2=int_{0}^{pi}W(omega)|E(e^{jomega})|^2domegatag{3}$$
with some positive weighting function $W(omega)$. Note that $varepsilon_1$ and $varepsilon_2$ given by $(2)$ and $(3)$ do not depend on frequency.
The authors of the paper you refer to chose to minimize the weighted mean square error given by $(3)$. However, instead of using the linear difference $(1)$, they chose to minimize the average logarithmic difference, i.e., the average error on a dB scale.
The problem with the minimization of $(3)$ is that for IIR filters, it results in a non-linear optimization problem, which is much harder to solve directly, and which might also have locally optimal solutions that are far from the global optimum. The cost function $hat{C}$ in your question is linear in the filter coefficients. The point of the iteration is now to solve a sequence of linear minimization problems (which is simple, just solve a system of linear equations) in order to compute the solution of the originally non-linear optimization problem (the minimization of $(3)$). Note that if convergence is achieved, then $A_{i-1}(e^{jomega})=A_{i}(e^{jomega})$, so the cost function $hat{C}_i$ is identical to the cost function of the original non-linear problem. Yet, only linear minimization problems are solved in each iteration.
I'm not sure I completely understand your last question, but the weighting function is there to change to problem from minimizing the average squared difference between frequency responses to the average squared differences between the logarithms of the magnitude responses. The necessary weighting function is unknown, but if the procedure converges - note that there is no guarantee that it does in all cases - the final weight function is such that the mean squared logarithmic error is minimized. Note that this latter problem is highly non-linear, whereas the proposed procedure only solves linear subproblems.
$endgroup$
$begingroup$
Thank you for your response! I now understand why the weighting is utilized. Could you elaborate on what is achieved by dividing by $A_{i-1}$ in the iterative cost function? Does it force convergence?
$endgroup$
– Jonas Schwarz
14 mins ago
$begingroup$
Also, i made an error in my first question, i meant to ask about the integral over the difference of true and estimated transfer function instead of a frequency-dependent error. I'll edit the question.
$endgroup$
– Jonas Schwarz
14 mins ago
add a comment |
$begingroup$
The chosen cost function is the mean squared error, i.e., the integral over a squared magnitude of the difference between frequency responses. The function
$$E(e^{jomega})=H(e^{jomega})-frac{B(e^{jomega})}{A(e^{j omega})}tag{1}$$
depends on frequency, so you can't minimize it directly, unless you want to minimize it for exactly one frequency $omega$, which is of course pointless. You can choose several error measures depending on $E(e^{jomega})$ given in $(1)$. Two common choices are
$$varepsilon_1=max_{omega}W(omega)|E(e^{jomega})|tag{2}$$
and
$$varepsilon_2=int_{0}^{pi}W(omega)|E(e^{jomega})|^2domegatag{3}$$
with some positive weighting function $W(omega)$. Note that $varepsilon_1$ and $varepsilon_2$ given by $(2)$ and $(3)$ do not depend on frequency.
The authors of the paper you refer to chose to minimize the weighted mean square error given by $(3)$. However, instead of using the linear difference $(1)$, they chose to minimize the average logarithmic difference, i.e., the average error on a dB scale.
The problem with the minimization of $(3)$ is that for IIR filters, it results in a non-linear optimization problem, which is much harder to solve directly, and which might also have locally optimal solutions that are far from the global optimum. The cost function $hat{C}$ in your question is linear in the filter coefficients. The point of the iteration is now to solve a sequence of linear minimization problems (which is simple, just solve a system of linear equations) in order to compute the solution of the originally non-linear optimization problem (the minimization of $(3)$). Note that if convergence is achieved, then $A_{i-1}(e^{jomega})=A_{i}(e^{jomega})$, so the cost function $hat{C}_i$ is identical to the cost function of the original non-linear problem. Yet, only linear minimization problems are solved in each iteration.
I'm not sure I completely understand your last question, but the weighting function is there to change to problem from minimizing the average squared difference between frequency responses to the average squared differences between the logarithms of the magnitude responses. The necessary weighting function is unknown, but if the procedure converges - note that there is no guarantee that it does in all cases - the final weight function is such that the mean squared logarithmic error is minimized. Note that this latter problem is highly non-linear, whereas the proposed procedure only solves linear subproblems.
$endgroup$
The chosen cost function is the mean squared error, i.e., the integral over a squared magnitude of the difference between frequency responses. The function
$$E(e^{jomega})=H(e^{jomega})-frac{B(e^{jomega})}{A(e^{j omega})}tag{1}$$
depends on frequency, so you can't minimize it directly, unless you want to minimize it for exactly one frequency $omega$, which is of course pointless. You can choose several error measures depending on $E(e^{jomega})$ given in $(1)$. Two common choices are
$$varepsilon_1=max_{omega}W(omega)|E(e^{jomega})|tag{2}$$
and
$$varepsilon_2=int_{0}^{pi}W(omega)|E(e^{jomega})|^2domegatag{3}$$
with some positive weighting function $W(omega)$. Note that $varepsilon_1$ and $varepsilon_2$ given by $(2)$ and $(3)$ do not depend on frequency.
The authors of the paper you refer to chose to minimize the weighted mean square error given by $(3)$. However, instead of using the linear difference $(1)$, they chose to minimize the average logarithmic difference, i.e., the average error on a dB scale.
The problem with the minimization of $(3)$ is that for IIR filters, it results in a non-linear optimization problem, which is much harder to solve directly, and which might also have locally optimal solutions that are far from the global optimum. The cost function $hat{C}$ in your question is linear in the filter coefficients. The point of the iteration is now to solve a sequence of linear minimization problems (which is simple, just solve a system of linear equations) in order to compute the solution of the originally non-linear optimization problem (the minimization of $(3)$). Note that if convergence is achieved, then $A_{i-1}(e^{jomega})=A_{i}(e^{jomega})$, so the cost function $hat{C}_i$ is identical to the cost function of the original non-linear problem. Yet, only linear minimization problems are solved in each iteration.
I'm not sure I completely understand your last question, but the weighting function is there to change to problem from minimizing the average squared difference between frequency responses to the average squared differences between the logarithms of the magnitude responses. The necessary weighting function is unknown, but if the procedure converges - note that there is no guarantee that it does in all cases - the final weight function is such that the mean squared logarithmic error is minimized. Note that this latter problem is highly non-linear, whereas the proposed procedure only solves linear subproblems.
answered 55 mins ago
Matt L.Matt L.
51.6k23994
51.6k23994
$begingroup$
Thank you for your response! I now understand why the weighting is utilized. Could you elaborate on what is achieved by dividing by $A_{i-1}$ in the iterative cost function? Does it force convergence?
$endgroup$
– Jonas Schwarz
14 mins ago
$begingroup$
Also, i made an error in my first question, i meant to ask about the integral over the difference of true and estimated transfer function instead of a frequency-dependent error. I'll edit the question.
$endgroup$
– Jonas Schwarz
14 mins ago
add a comment |
$begingroup$
Thank you for your response! I now understand why the weighting is utilized. Could you elaborate on what is achieved by dividing by $A_{i-1}$ in the iterative cost function? Does it force convergence?
$endgroup$
– Jonas Schwarz
14 mins ago
$begingroup$
Also, i made an error in my first question, i meant to ask about the integral over the difference of true and estimated transfer function instead of a frequency-dependent error. I'll edit the question.
$endgroup$
– Jonas Schwarz
14 mins ago
$begingroup$
Thank you for your response! I now understand why the weighting is utilized. Could you elaborate on what is achieved by dividing by $A_{i-1}$ in the iterative cost function? Does it force convergence?
$endgroup$
– Jonas Schwarz
14 mins ago
$begingroup$
Thank you for your response! I now understand why the weighting is utilized. Could you elaborate on what is achieved by dividing by $A_{i-1}$ in the iterative cost function? Does it force convergence?
$endgroup$
– Jonas Schwarz
14 mins ago
$begingroup$
Also, i made an error in my first question, i meant to ask about the integral over the difference of true and estimated transfer function instead of a frequency-dependent error. I'll edit the question.
$endgroup$
– Jonas Schwarz
14 mins ago
$begingroup$
Also, i made an error in my first question, i meant to ask about the integral over the difference of true and estimated transfer function instead of a frequency-dependent error. I'll edit the question.
$endgroup$
– Jonas Schwarz
14 mins ago
add a comment |
Thanks for contributing an answer to Signal Processing Stack Exchange!
- Please be sure to answer the question. Provide details and share your research!
But avoid …
- Asking for help, clarification, or responding to other answers.
- Making statements based on opinion; back them up with references or personal experience.
Use MathJax to format equations. MathJax reference.
To learn more, see our tips on writing great answers.
Sign up or log in
StackExchange.ready(function () {
StackExchange.helpers.onClickDraftSave('#login-link');
});
Sign up using Google
Sign up using Facebook
Sign up using Email and Password
Post as a guest
Required, but never shown
StackExchange.ready(
function () {
StackExchange.openid.initPostLogin('.new-post-login', 'https%3a%2f%2fdsp.stackexchange.com%2fquestions%2f56860%2fcost-function-for-lti-system-identification%23new-answer', 'question_page');
}
);
Post as a guest
Required, but never shown
Sign up or log in
StackExchange.ready(function () {
StackExchange.helpers.onClickDraftSave('#login-link');
});
Sign up using Google
Sign up using Facebook
Sign up using Email and Password
Post as a guest
Required, but never shown
Sign up or log in
StackExchange.ready(function () {
StackExchange.helpers.onClickDraftSave('#login-link');
});
Sign up using Google
Sign up using Facebook
Sign up using Email and Password
Post as a guest
Required, but never shown
Sign up or log in
StackExchange.ready(function () {
StackExchange.helpers.onClickDraftSave('#login-link');
});
Sign up using Google
Sign up using Facebook
Sign up using Email and Password
Sign up using Google
Sign up using Facebook
Sign up using Email and Password
Post as a guest
Required, but never shown
Required, but never shown
Required, but never shown
Required, but never shown
Required, but never shown
Required, but never shown
Required, but never shown
Required, but never shown
Required, but never shown
BXVLKE0oj